The Transformative Power of Healthcare Datasets for Machine Learning in Business
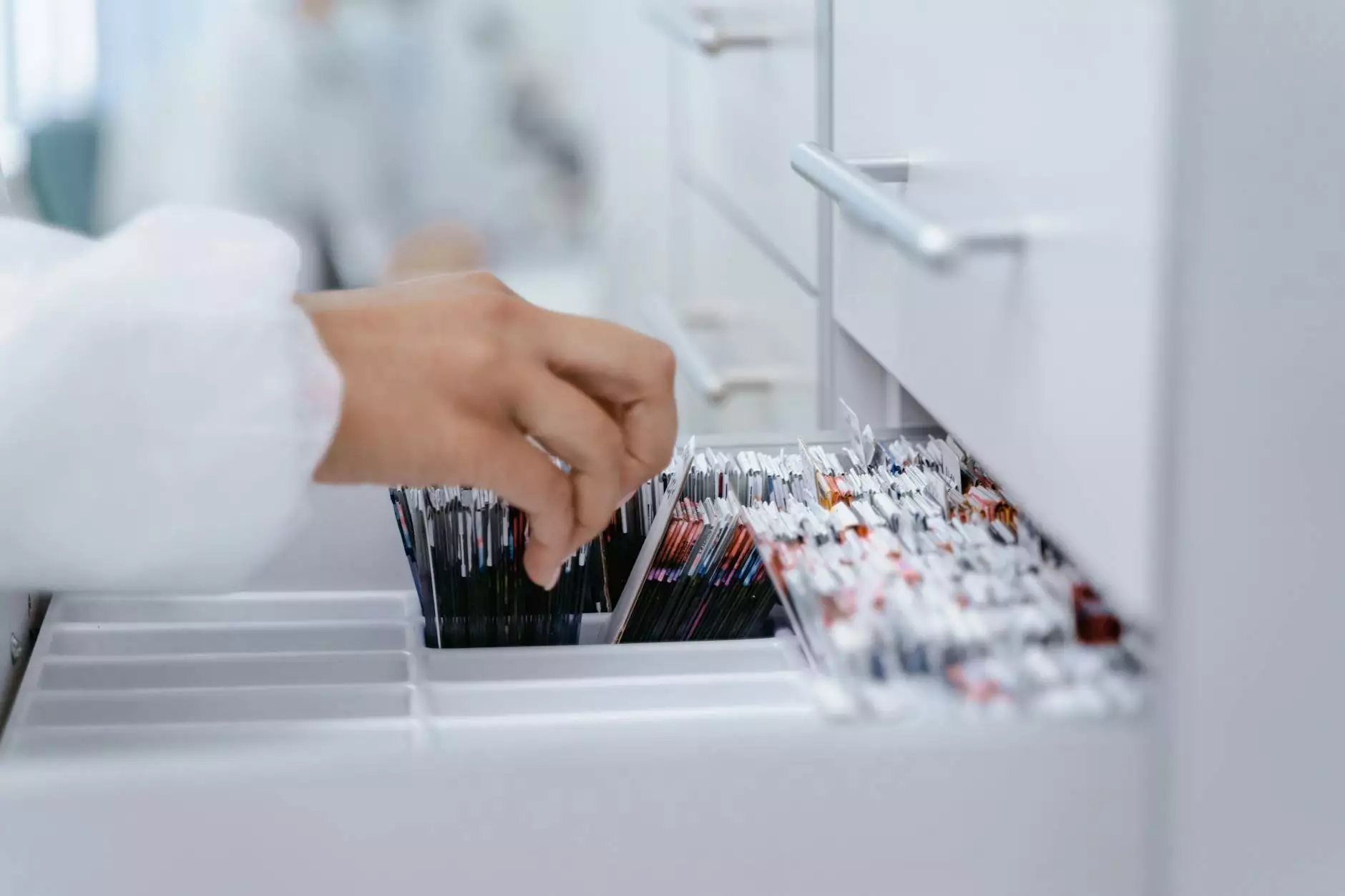
Healthcare datasets for machine learning represent a revolutionary leap in how businesses can harness data to drive decisions, improve efficiency, and enhance services. In an era where data reigns supreme, the ability to extract actionable insights from vast quantities of information is not just advantageous; it is essential for survival in the competitive landscape.
Understanding Healthcare Datasets
Healthcare datasets encompass a plethora of information including, but not limited to:
- Patient demographics - Details such as age, gender, and socioeconomic status.
- Clinical data - Information gathered from medical records, treatments, diagnoses, and outcomes.
- Financial data - Insights into costs, billing, and revenue related to healthcare services.
- Operational data - Data regarding the functioning of healthcare facilities, including patient throughput and staff efficiency.
- Public health data - Statistics related to population health, disease prevalence, and health behaviors that impact communities.
Why is Machine Learning Essential for Healthcare Datasets?
Machine learning (ML) enhances the utility of datasets by enabling systems to learn from data, identify patterns, and make predictions with minimal human intervention. Here’s why integrating healthcare datasets for machine learning into business practices is a game-changer:
1. Improving Patient Outcomes
Using ML algorithms, healthcare providers can analyze patient data to predict health outcomes, facilitating early interventions. For instance, predictive analytics can identify patients at high risk of chronic diseases, enabling preemptive care measures.
2. Enhancing Operational Efficiency
Businesses can use ML to streamline operations by analyzing workflow patterns and identifying inefficiencies. This can lead to better resource allocation, reduced wait times, and overall improved patient satisfaction.
3. Personalizing Patient Care
With the help of ML, healthcare providers can offer tailored treatment plans based on individual patient data. This personalization increases the effectiveness of treatments and fosters patient loyalty.
4. Optimizing Financial Management
Organizations can leverage ML to analyze financial datasets and identify trends, potential revenue losses, or unnecessary expenditures. This data-driven decision-making leads to improved financial health for healthcare businesses.
Challenges in Utilizing Healthcare Datasets
While the benefits are substantial, integrating healthcare datasets for machine learning comes with its challenges:
- Data Privacy Concerns - Healthcare data is sensitive and subject to strict regulations. Ensuring compliance (such as HIPAA in the United States) while utilizing this data is critical.
- Data Quality - The accuracy of machine learning models is heavily dependent on the quality of the data. Incomplete or erroneous data can lead to misleading outcomes.
- Integration Issues - Merging various datasets from different sources can be complex and resource-intensive, requiring significant time and expertise.
- Skills Gap - There is often a lack of skilled personnel who can correctly interpret and analyze healthcare data effectively.
Best Practices for Leveraging Healthcare Datasets for Machine Learning
To effectively utilize healthcare datasets for machine learning, businesses should adhere to several best practices:
1. Ensuring Data Compliance
Staying compliant with data protection regulations is non-negotiable. Businesses should implement robust data governance policies that ensure the ethical use of healthcare data.
2. Data Cleaning and Preprocessing
A robust data preprocessing routine is crucial. This includes removing duplicates, handling missing values, and ensuring consistent formatting. Clean data improves the accuracy of machine learning models.
3. Adopting a Patient-Centric Approach
Healthcare is predominantly service-oriented. Keeping the patient experience at the forefront while analyzing data ensures that ML applications foster well-being and satisfaction.
4. Fostering Collaboration with Data Scientists
Healthcare organizations should work alongside data science professionals who can navigate the intricacies of machine learning, ensuring that insights generated from healthcare datasets are actionable and beneficial.
Applications of Machine Learning in Healthcare
The applications of machine learning powered by healthcare datasets for machine learning are vast and varied:
1. Disease Prediction and Diagnosis
Machine learning models can analyze patient data to predict the likelihood of diseases, helping physicians make timely diagnoses and overall improve patient outcomes.
2. Treatment Recommendation Systems
These systems analyze historical patient data to provide healthcare providers with the best treatment protocols for individual patients based on similar cases.
3. Medical Imaging Analysis
ML algorithms can enhance the analysis of medical images, leading to quicker and more accurate diagnoses. For example, they can identify abnormalities in x-rays or MRIs that might be overlooked by the human eye.
4. Administrative Workflow Automation
ML can automate administrative tasks such as appointment scheduling, patient follow-ups, and insurance verification processes, allowing healthcare organizations to focus on patient care.
5. Drug Discovery and Development
Machine learning can significantly accelerate the process of drug discovery by predicting how different compounds will perform based on historical datasets.
Future Trends in Healthcare Machine Learning
The landscape of healthcare datasets for machine learning is continuously evolving. Here are some expected trends in the near future:
1. Increased Use of Real-Time Data
With the rise of wearable technology and IoT devices, healthcare datasets will increasingly rely on real-time data to make immediate, informed decisions about patient care.
2. Enhanced Data Interoperability
Efforts to standardize healthcare data formats will improve collaboration across entities, enabling richer datasets for machine learning applications.
3. Expansion of Telehealth Services
As telehealth becomes more prevalent, the integration of ML will help optimize virtual care delivery, emphasizing patient engagement and outcomes.
4. Greater Focus on Predictive Analytics
Organizations will place more emphasis on utilizing predictive analytics to not only react to issues but to proactively address potential healthcare problems before they arise.
Conclusion
In conclusion, healthcare datasets for machine learning represent an invaluable asset for businesses in the healthcare sector. By harnessing the power of data analytics, organizations can significantly enhance patient care, improve operational efficiency, and drive financial sustainability. As we move forward, adopting best practices and staying abreast of emerging trends will be essential for organizations looking to leverage these datasets effectively. The future of healthcare will increasingly be defined by data-driven decisions, making machine learning an indispensable tool for success.